Instagram reveals the truth behind how its algorithm sorts content
The new Recommended posts we see on our Instagram feeds are picked out by an AI algorithm, explains the company
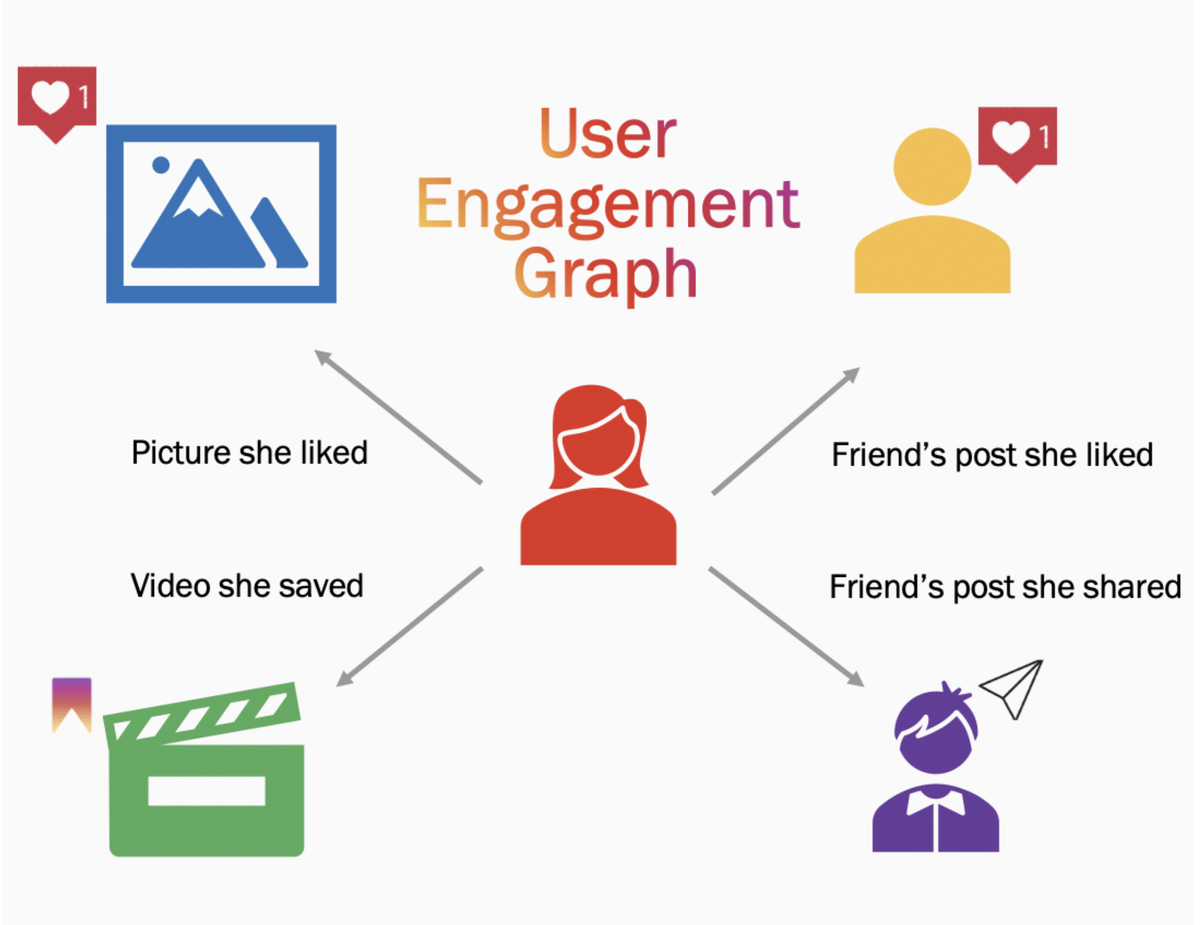
The engineering team at Instagram has published an explainer revealing how the platform's AI algorithm works, using information retrieval systems to dictate which new Suggested and Recommended posts appear in our social media feeds.
Instagram has recently backtracked on a few of its planned updates, as a result of being under fire from unhappy users (with even the Kardashians taking a stand) fed up from seeing too many suggested posts and not enough from their friends.
• Looking for the best cameras for Instagram?
We've reported a lot recently on how Instagram is no longer fit for photographers, with the platform making it very clear that Reels and (TikTok-inspired) video content are now its top priority.
Instagram is also on pretty thin ice after receiving bad press for banning a cosplayer, as well as the spark of a Make Instagram Instagram again movement that involved celebrities and influencers coming together to rally against the algorithm. Maybe Instagram needs a PR cleanup and would benefit from a workshop on how not to infuriate its users – with many switching over to BeReal instead.
The team over at Meta Engineering have recently published an article explaining how Instagram suggests new content, that goes into some pretty in-depth details on the inner workings of the AI – which relies on what it calls a Home Feed Ranking System and an Explore Ranking System to sort posts from those that you follow, as well as other public posts that might be relevant and engaging to you.
Instagram says that users who remain engaged are the ones that keep finding newer sources of interests to follow, and this works by algorithm and ranking models that make judgements based on factors like engagement, relevance, and freshness. Basically the platform uses information from posts that you like, comment, save or engage with to determine what might interest you, as well in some cases as the posts that your friends like and engage with too.
Get the Digital Camera World Newsletter
The best camera deals, reviews, product advice, and unmissable photography news, direct to your inbox!
The Suggested Posts feature was launched in August 2020 to achieve the objective of showing new posts to users from accounts that they do not follow, yet perhaps feel like they discovered all on their own. These are the types of posts that currently show up at the end of our feeds, after we run out of content from those we follow.
"Scrolling through the End of Feed Recommendations should feel like scrolling down an extension of Instagram Home Feed," says Amogh Mahapatra, Meta Machine Learning Engineer and author of the published article.
There's more technicalities to this information retrieval process. The system it uses for recommending posts apparently has a two-step design: candidate generation and candidate selection. A candidate is either something (a post) or someone (a user) that another user could possibly be interested in, based on their Instagram activity that reveals their own explicit or implicit interests, and this stage is what the company calls a recall-heavy stage.
The second stage of candidate selection involves a typically heavier weighted ranking algorithm, selecting the best subset (end result, Reel, or post) from a selection that is then finally shown to the user. The design of the Suggested Posts ranking system, however, is a little different, with post recommendations falling under two separate categories: Connected or Unconnected. Connected recommendations are posts from accounts that the user actually follows, ranked based on engagement.
The company demonstrates the workings of this design structure through a flow-chart (below) explaining that an Unconnected recommendation system, such as Suggested Posts, derives sources based on a user's activity across Instagram rather than posts from followed users, though it ranks posts based on similar factors.
"A user’s activities on Instagram helps us in building a virtual graph of their interests," says the platform. Account embeddings also assist the platform in finding accounts that are thematically and topically similar to one another.
Things get a little more obscure when the article begins to communicate in metaphors using "seeds" to describe what is an author or media of interest to a user. These seeds of interests can then develop into K-nearest neighbor (KNN) pipelines, outputting similar content as the original seeds. These "pipelines" are created and based around two principles that involve similar 'account embeddings', comparable to word embedding vectors, and 'co-occurrence' based similarity.
In simple terms, the AI uses pattern-mining and user-media interaction data such as liking a post of a certain genre, in the co-occurence principle, to find frequencies of media (seeds) to generate similar recommendations to users.
The company has identified what it calls a cold start problem, where some users may not have enough engagement or activity to draw from in generating an inventory of candidates (posts) for suggestion. This is dealt with by using a fallback graph exploration approach by evaluating a user's connections – so accounts followed by this account in a chain, for consideration for use as a seed.
The second method of resolving a cold start, particularly for extremely new users of the platform, is to suggest generic popular media posts, and then adapting parameters based on a users response to these popular media items to build from. The article goes into greater detail on the exact terminology and processes continuing on from this, so it's best to read the Meta Engineering piece to fully grasp these concepts for those interested.
It's certainly an eye-opener to think about and understand how every single aspect of our data and what we do on social platforms is used to essentially generate a digital marketing profile for us – controlling everything from adverts, sponsored posts and recommended Reels based on what an AI thinks we want to see.
• You may also be interested in learning how to use Instagram Reels, or perhaps you need to know How to use Instagram filters, and understand the best way to organise your grid with similar content.
A staff writer for Digital Camera World, Beth has an extensive background in various elements of technology with five years of experience working as a tester and sales assistant for CeX. After completing a degree in Music Journalism, followed by obtaining a Master's degree in Photography awarded by the University of Brighton, she spends her time outside of DCW as a freelance photographer specialising in live music events and band press shots under the alias 'bethshootsbands'.