What can videogame developers AI teach wildlife cameras? "Equal representation"
There may not be enough images of rare species for AI to idntify them on trail cameras, so why not create them?
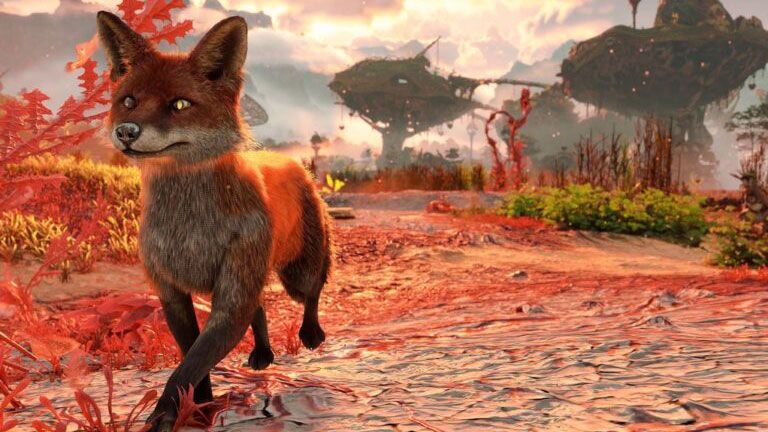
Artificial intelligence programs for camera trap image recognition have become pretty good at identifying commonly seen wildlife, but they struggle with rare species due to a lack of available learning materials.
To tackle this issue, one scientist believes that the future of AI recognition of rare animals lies in the gaming world.
Before AI can even begin to differentiate between a badger or a deer, it needs to be taught their individual profiles using imagery. The AI will struggle, however, if very few images of said species exist due to its rarity. Current camera trap image recognition AI can spot a raccoon from just a flick of its tail, but it can’t pick out an endangered kit fox staring it right in the face.
In an article on conservation website Mongabay, biologist Zhengyang Wang explains that a few years ago he was catching butterflies in a strip of rainforest, when “a team of jet-lagged vagabonds dragging their rollers full of flashlights and 3D scanners” arrived.
Initially thinking they were a film crew, he quickly realized that they were developers from a well-known game studio there to capture details of the natural world for their next big open-world videogame – a type of game that enables players to explore and interact with a roamable, virtual world.
“Increasingly I have also observed that tools used by game developers are helping real-world conservationists,” he writes.
As it happened, AI doesn’t care whether it’s learning from real-life images or engineered hyper-realistic animal images. He references a colleague’s mission to improve her AI’s ability to detect deer in camera trap images, taken across the southwestern United States where sightings are rare.
Get the Digital Camera World Newsletter
The best camera deals, reviews, product advice, and unmissable photography news, direct to your inbox!
“Her team simulated a forest environment in Unity [a widely used game engine] and loaded 17 digital deer models created by other game developers. They took thousands of 'photos' of digital deer roaming the digital forest, and used these images to train their AI.
“Feeding the AI images of these 'synthetic deer' increased its deer detection rate by 40% – that is a huge win for finding more deer, at the minimum cost of using pre-developed environments and pre-modeled deer.”
Wang believes that this is more about "equal representation" than it is just detecting deer.
“We often hear about how AI is drastically changing the landscape of conservation biology, but conservationists have these synthetic beasts and their virtual habitats to thank for making sure our AI is not ignoring the rarely seen and the underrepresented: the dipteran pollinators, the cave-dwelling bats, the crawlers and creepers – they matter in their intrinsic biodiversity value.”
Interested in wildlife? Why not check out our guides to the best cameras for wildlife photography, the best trail cameras, and the best cheap drones.
After graduating from Cardiff University with an Master's Degree in Journalism, Media and Communications Leonie developed a love of photography after taking a year out to travel around the world.
While visiting countries such as Mongolia, Kazakhstan, Bangladesh and Ukraine with her trusty Nikon, Leonie learned how to capture the beauty of these inspiring places, and her photography has accompanied her various freelance travel features.
As well as travel photography Leonie also has a passion for wildlife photography both in the UK and abroad.